In the Jisc AI team we spend a lot of our time exploring different AI tools and services, assessing their potential to enhance education. With that in mind we are launching Product Notes, a new blog series, to share our knowledge and findings with all of our members. In each entry in this series, we’ll be looking in-depth at a particular AI tool or service with a focus on education, we’ll explore their potential for education and where possible we’ll be talking to institutions currently using these tools to share their experiences too.
Our very first Product Note below looks at Explorance MLY, a platform which utilises AI for qualitative analysis using machine learning models made bespoke for analysing the student experience.
About MLY
Explorance offer a range of solutions for evaluation and survey management and analytics, some institutions may be familiar with their feedback analytics platform Explorance Blue for example.
We have looked specifically though at Explorance MLY, their AI assisted analysis platform which enables users to run analysis of their qualitative data through one of three models: Student Experience Insights (SEI), Employee Learning Insights (ELI) and Employee Experience Insight (EEI). We focused on the SEI model when we looked at MLY, but it’s worth noting that access to the other two models is included and allows for analysis of employee feedback too.
Users import their own data into MLY in either Excel or CSV format, once uploaded they identify the columns in which the qualitative data sits and apply the desired analysis type (in our case SEI). Explorance do not access or use this data and it won’t be utilised in the training of their models unless institutions consent to sharing their data for that purpose. The SEI model has an extensive range of topics and subtopics which the model will apply to the qualitative feedback, these aim to cover all aspects of the student experience including course components, expenses, remote learning and more. The SEI topic list can be explored in full here.
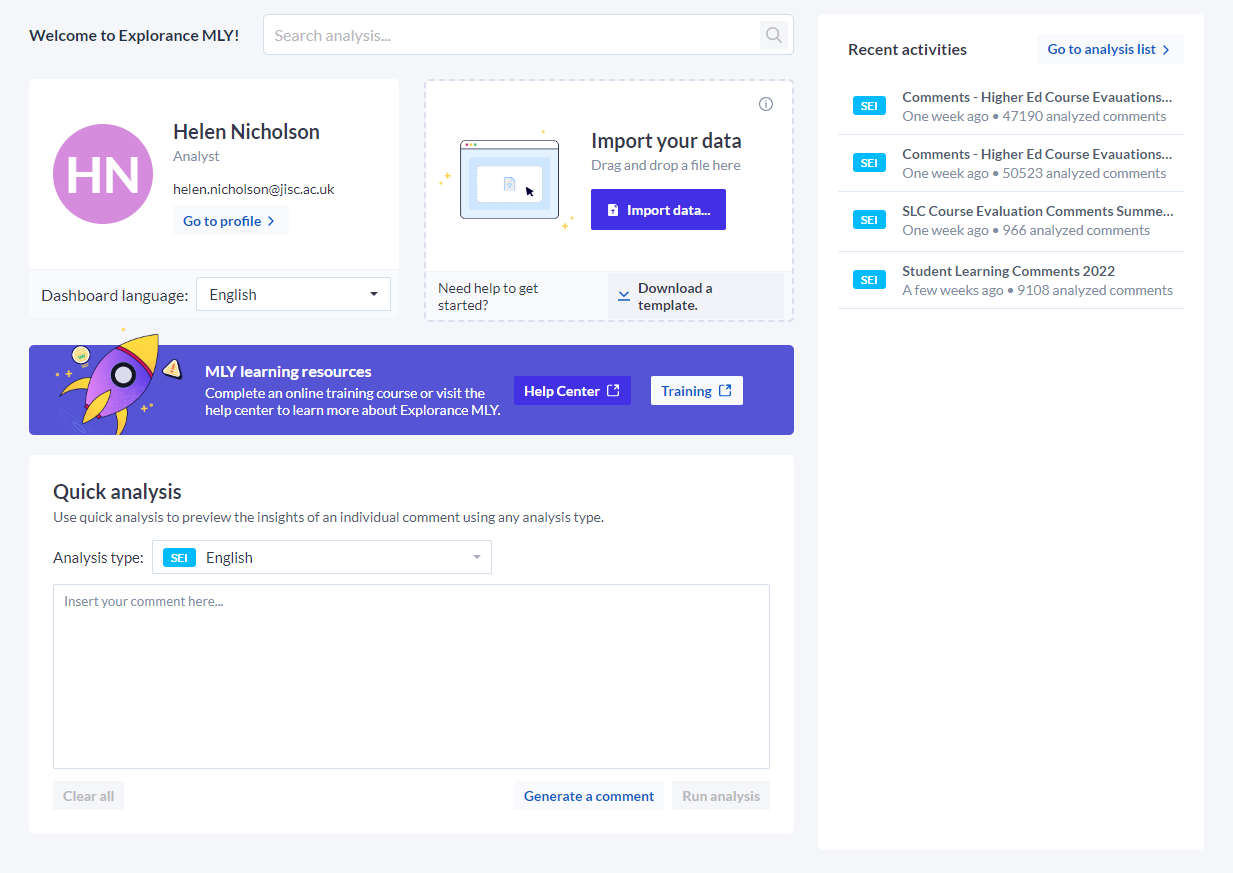
Notably, users can also run analysis without applying one of the tailored models. The standard analysis always includes:
- Sentiment analysis: Determines the overall sentiment of a comment and marks them as positive, negative, neutral, not explicit or mixed.
- Recommendations: Labels comments with a potential action based on the content of a comment, the actions are “Do more”, “Do Less”, “Start”, “Stop”, “Continue”, or “Change”.
- Alerts: Highlights comments which contain concerning content and may require immediate action.
Once the analysis is completed the user can access a comprehensive dashboard to explore their data from. This dashboard offers a summary of sentiment percentages, recommendations, and alerts. Users can delve deeper through the Topic and Comment explorer sections, filterable by the SEI topic framework and demographic/category data. Comments can be further sorted by sentiment, recommendation quantity, or alert quantity.
The analysis can also be shared to other users within Explorance or exported back to a CSV or Excel file for easy sharing.
Key points:
It’s very fast:
The speed at which MLY can analyse data is one of its key benefits. We ran several files through the platform with sizes varying from 900 to over 50,000 rows of data. Smaller files completed in less than a few minutes and even the largest file took no more than 30 minutes to process. Compared to traditional methods for manually categorising data the time saving potential is considerable.
Features help guide users to actionable insights:
MLY has some particularly useful features which support users with getting into the analysis of their feedback and ascertaining actions to take quickly.
The alerts system provides a way to quickly identify areas of concern and was effective in our tests at flagging comments which met the criteria. Helpfully, users can also adjust the threshold % for alerts to widen or restrict the alert score needed to raise a flag.
We found the combination of filters and sorting available in the analysis dashboard made it easy to locate comments on specific topics quickly. For instance, a user looking for feedback to inform improvements to library facilities can quickly view all negative comments about library facilities by applying the relevant topic and sentiment filters.
The widgets feature is very helpful here too, allowing users to create and save preferred combinations of filters within the dashboard – preset widgets are also provided to show highlights including most frequent topics, most positive and areas for improvement based on frequency of negative comments.
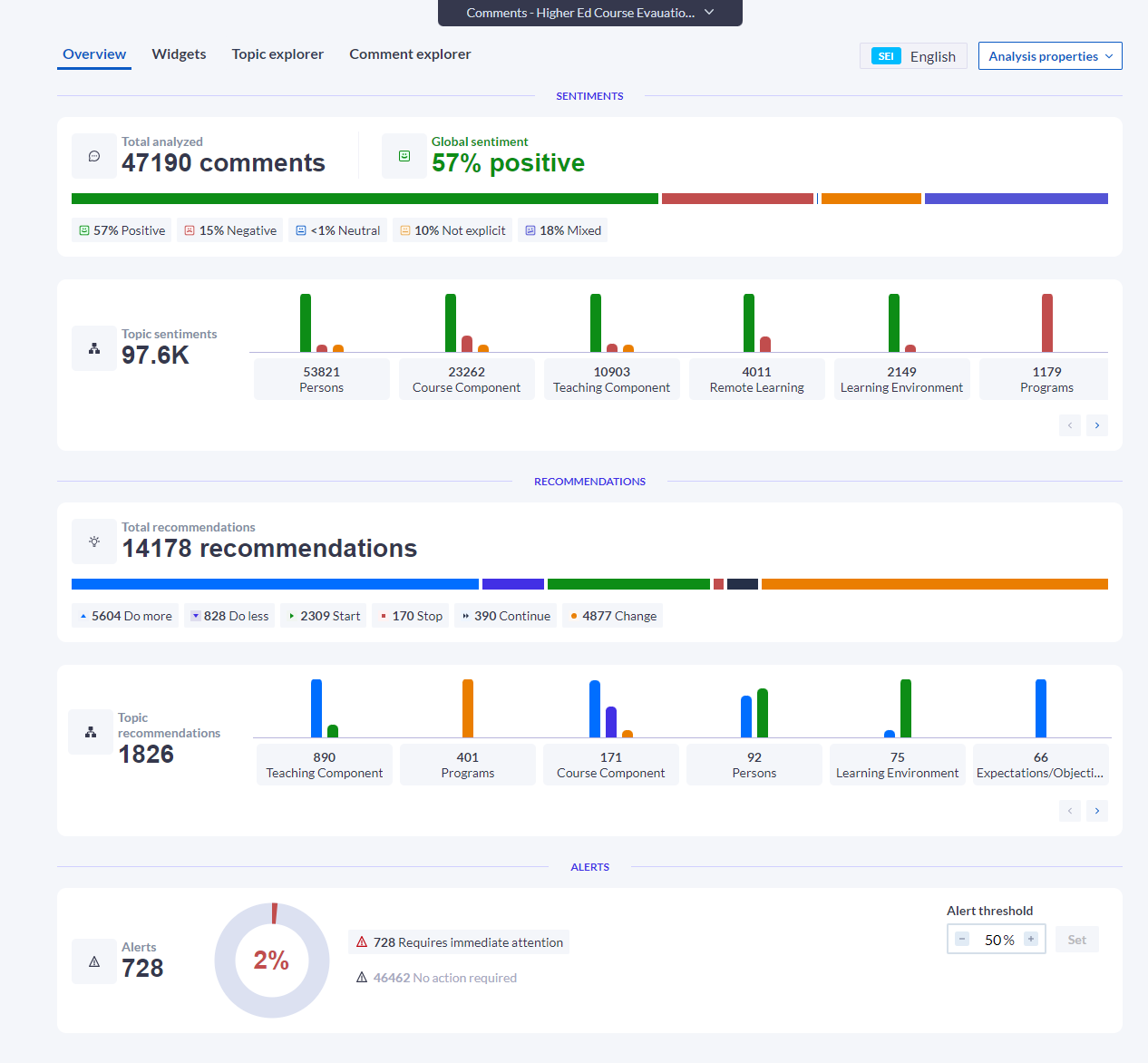
Easy to use:
MLY is straightforward to get started with, as the importing of data is left to the user there is minimal setup required. The platform is very flexible and can be used to analyse data from a variety of sources including large scale surveys like the NSS to end of module evaluations.
Overall, we found the platform itself very easy to use and navigate. The interface is simple, and we got the hang of navigating the dashboard quickly. There is a help-centre section too with supporting articles, and the Explorance team run regular training webinars.
We particularly liked the ‘quick analysis’ feature also which allows users to paste individual comments into a section on the MLY homepage for a quick analysis rather than having to upload a file with a small amount of data.
Lots of options for sharing results:
Lastly, the platform makes it easy to quickly export the completed analysis back into a csv or excel file which means results can be shared without requiring all members of staff to access the platform directly. Both bases are covered though as the in-platform sharing system and ability to create ‘guest users’ makes it possible to share completed analyses within the platform too.
Like all such systems, it won’t categorise all comments:
We found that there was some variation of the % of comments which are categorised by MLY. In running our files, we experienced a range of up to 25% of comments not being applied a category from the SEI topics list.
Some of this will be the result of comments which do not present enough information to categorise e.g., extremely short comments of one or two words. Notably, uncategorised comments still had the sentiment, alerts and recommendations analysis applied to them so there was still some analysis provided.
We expect that the percentage of comments which go uncategorised should improve as MLY’s models are improved through feedback from their users.
Since we first began looking at MLY only a few months ago they have made significant developments to their platform, and we expect it will only continue to improve.
From a current user…
We spoke to current users of MLY at Liverpool John Moores University to learn about their experience of the tool:
“We recently introduced MLY into our routine analysis of student feedback. The platform allows us to quickly identify insights and trends from large data sets, and compare student views across demographic groups. Particularly useful features include alerts that highlight areas for immediate attention and action categories (e.g. ‘do more/less’, ‘continue’ and ‘stop’) that suggest foci for activity. A new sharing feature will facilitate the decentralisation of analysis to support local investigation and insight. MLY’s student experience insights model provides a generally reliable categorisation structure for UK universities, and we anticipate that this will improve as the system learns from a growing UK dataset. We are encouraged to contribute to this by providing feedback to the MLY development team. Explorance offer excellent customer support and invest in developing a community of users. This provides valuable opportunities to share good practice in the use of MLY, as well as wider issues associated with student feedback.”
-Dr Elena Zaitseva, Head of Student Voice and Evaluation, and Dr Phil Carey, Dean of Teaching and Learning Academy, Liverpool John Moores University
(Most) Suitable for
MLY could be a good solution if your institution:
- Collects large quantities of qualitative student feedback
- Is looking to improve its use of qualitative student feedback
- Wants to reduce the time/effort spent processing that data
- Wants to respond to feedback/ascertain actions quickly
At this time MLY is probably a solution that will be more suited to higher education institutions as it is more appropriate for those analysing very large quantities of data and may not be as cost-effective for FE. Though this of course depends on individual institutions’ circumstances.
Pricing
MLY is available on an annual subscription and pricing is based on the student FTE number. Discounts are available for multi-year contracts. To enquire about pricing for your institution contact Explorance using the details below.
To find out more
Get in touch with the Explorance team to find out more about MLY and arrange a demo to find out whether it is a suitable solution for your needs:
Website: explorance.com
Contact: John Atherton, VP Sales
Email: jatherton@explorance.com
Phone: 07764481205
Find out more by visiting our Artificial Intelligence page to view publications and resources, join us for events and discover what AI has to offer through our range of interactive online demos.
For regular updates from the team sign up to our mailing list.
Get in touch with the team directly at ai@jisc.ac.uk
2 replies on “Product Notes – Explorance MLY ”
Do you have other product notes as suggested? And a matrix of features for each product would be useful.
Hi Andy, yes we have now added a couple more Product Notes entries over the last few weeks covering Tutello and Blackboard Learn Ultra AI Design Assistant – you can find these in the Product Notes category (https://nationalcentreforai.jiscinvolve.org/wp/category/product-notes/). We’ll continue adding more entries as we investigate more products.
Thank you also for your suggestion of a matrix, we are exploring different ways to share product/tool information and this is helpful to consider.